
Speaker "Yunsong Guo" Details Back
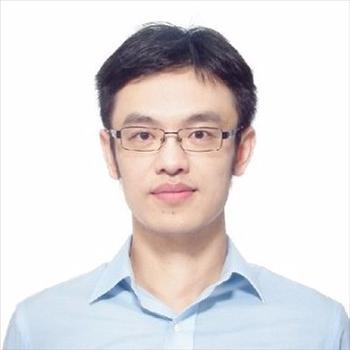
-
Name
Yunsong Guo
-
Company
Pinterest
-
Designation
Staff Software Engineer
Topic
How Pinterest Uses Machine Learning to Achieve ~200M Monthly Active Users
Abstract
Being valued at 12B USD, Pinterest has always prioritized user experiences over everything else. Since 3 years ago we started to use machine learning in our most important product, i.e.,Pinterest Homefeed. The series of machine learning models we launched over these years, including linear models, Gradient Boosted Decision Trees (GBDT) models and deep neural network models have dramatically increased both the number of active users on Pinterest and the level of user engagement, and we are now close to 200M monthly active users. Yunsong is a founding member of the Pinterest Homefeed ranking team, and is excited to share with the audience details of the evolution of Pinterest Homefeed machine learned recommendation models over the past 3.5 years. In particular, this talk includes 3 main parts: introduction of machine learning in various Pinterest products; how linear, GBDT and deep NN models are applied in Homefeed to improve user engagement, and practical lessons on finding the most impactful features.
Profile
Yunsong Guo is a staff software engineer at Pinterest, with expertise in machine learning and feature engineering based on large scale data analysis. He is a founding member of Pinterest Homefeed Ranking team, and is responsible for researching and developing machine learned ranking models for Homefeed recommendations. The series of models Yunsong deployed online over the past 3-4 years have increased Homefeed user engagement multifolds, directly contributed to Pinterest’s ~200M MAUs. Prior to Pinterest, Yunsong obtained his PhD from the Computer Science department of Cornell University, and worked as a quantitative researcher at Goldman Sachs London and Citadel Hong Kong on algorithmic trading and high-frequency trading.