
Speaker "Amir Meimand" Details Back
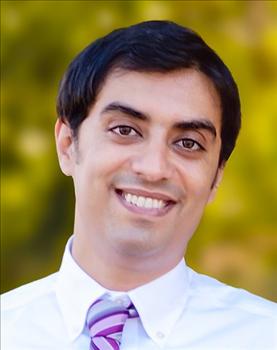
-
Name
Amir Meimand
-
Company
Zilliant
-
Designation
Director, Data Science
Topic
An Efficient ML-Based Algorithm for Mining Substitute Products for E-Commerce
Abstract
In design the recommendations system for retailers and suppliers it is very important to understand the relationship between products based on customer behavior. In general, if two products are related they can be either substituted or complementary. Extracting both complimentary and substitute products provides valuable knowledge for market prediction. Complementary rules mining by discovering the association rules and it is goal is to identify the products are purchased together.
There are considerable amount of efforts in the literature to improve the efficient and effectiveness of association rules mining. Substitute rules mining are mostly based on negative association rules mining. There are two main difficulties in recognizing substitute products using negative association rules. Firstly, mining negative association rules are computationally very expensive. Moreover, negative association rules usually generate a lot of redundant rules and needs.
In this talk we introduce an innovative approach to discover substitute product by deriving the similarities of products based on corresponding association rules. The proposed method is computationally efficient and effective. We will present a case study to demonstrate the application of proposed method in B2B e-commerce business