
Speaker "Emad Barsoum" Details Back
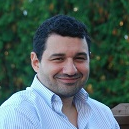

-
Name
Emad Barsoum
-
Company
Microsoft
-
Designation
Principal Architect
Topic
Overview of GAN based training and its applications.
Abstract
Profile
Emad Barsoum is an Architect at Microsoft AI Platform team. He leads the deep learning framework effort at Microsoft and help driving Microsoft strategy in AI. Prior to that Emad was Principal SDE and Applied Researcher in the Advance Technology Group at Microsoft Research. He was one of the core developer and researcher behind the Emotion Recognition algorithm used in MS Cognitive Service for both still image and video. Before that, He was one of the main Architects for NUI API on Xbox One, and the tech lead for the depth reconstruction pipeline for Kinect v2. His current research focuses are in computer vision and deep learning algorithms, especially in the area of activity detection/recognition and unsupervised learning. He has given numerous internal and external talks on Deep Learning and Computer Vision. He received his M.S. degree from U.C.Irvine and his doctoral degree from Columbia University.