
Speaker "Nima Safaei" Details Back
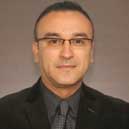

-
Name
Nima Safaei
-
Company
Scotiabank
-
Designation
Sr. Data Scientist
Topic
Regularization and False Alarms Quantification: Two Sides of the Explainability Coin
Abstract
Regularization is a well-established technique in machine learning (ML) to achieve an optimal bias-variance trade-off which in turn reduces model complexity and enhances explainability. To this end, some hyper-parameters must be tuned, enabling the ML model to accurately fit the unseen data as well as the seen data. In this article, the authors argue that the regularization of hyper-parameters and quantification of costs and risks of false alarms are in reality two sides of the same coin, explainability. Incorrect or non-existent estimation of either quantities undermines the measurability of the economic value of using ML, to the extent that might make it practically useless.
Prerequisite knowledge:
Learning Theory, Machine Learning, Analytics
Profile
Nima Safaei has a Ph.D. in system and industrial engineering with a background in Applied Mathematics. He held a postdoctoral position at C-MORE Lab (Center for Maintenance Optimization & Reliability Engineering), University of Toronto, Canada, working on various maintenance planning and scheduling problems in collaboration with ArcelorMittal, UK Ministry of Defence, and Hydro One Networks. He was with Department of Maintenance Support and Planning, Bombardier Aerospace with a focus on Operations Research & Machine Learning methods for reliability analysis and maintenance optimization. He is currently with Data Science & Analytics (DSA) lab, Bank of Nova Scotia, Toronto, Canada, as Senior Data Scientist.